Incremental processing is one of the core values provided by CocoIndex.
In CocoIndex, users declare the transformation, and don't need to worry about the work to keep index and source in sync.
CocoIndex creates & maintains an index, and keeps the derived index up to date based on source updates, with minimal computation and changes. That makes it suitable for ETL/RAG or any transformation tasks that stay low latency between source and index updates, and also minimizes the computation cost.
If you like our work, it would mean a lot to us if you could support Cocoindex on Github with a star. Thank you so much with a warm coconut hug 🥥🤗.
What is incremental processing?​
Figuring out what exactly needs to be updated, and only updating that without having to recompute everything throughout.
How does it work?​
You don't really need to do anything special, just focus on defining the transformation needed.
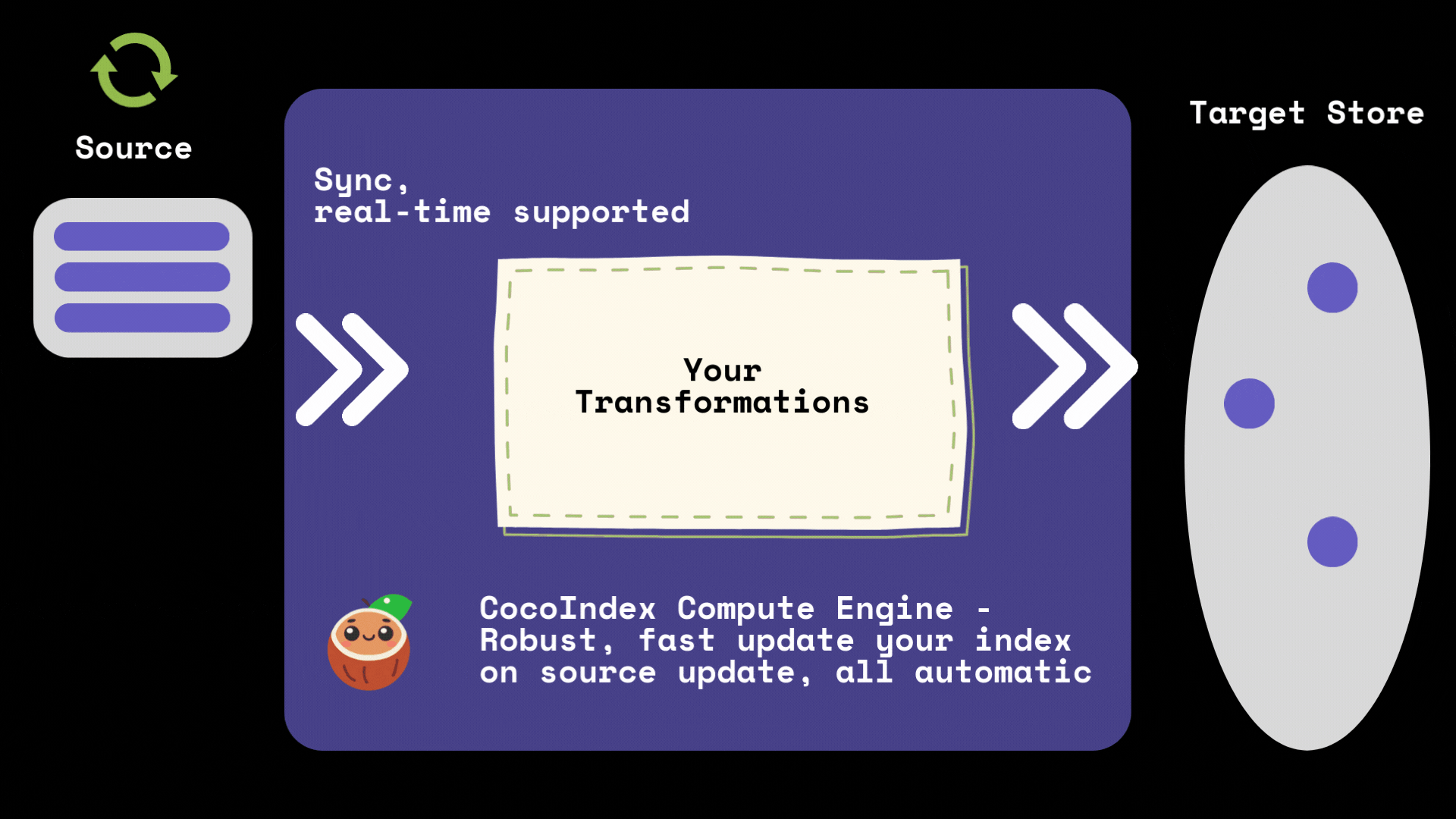
CocoIndex automatically tracks the lineage of your data and maintains a cache of computation results. When you update your source data, CocoIndex will:
- Identify which parts of the data have changed
- Only recompute transformations for the changed data
- Reuse cached results for unchanged data
- Update the index with minimal changes
And CocoIndex will handle the incremental processing for you.
CocoIndex provides two modes to run pipeline:
- One time update: Once triggered, CocoIndex updates the target data to reflect the version of source data up to the current moment.
- Live update: CocoIndex continuously reacts to changes of source data and updates the target data accordingly, based on various change capture mechanisms for the source.
Both modes run with incremental processing. You can view more details in Life Cycle of an Indexing Flow.
Who needs Incremental Processing?​
Many people may think incremental processing are only beneficial for large scale data. Thinking carefully,
it really depends on the cost and requirement for data freshness.
Google processes huge scale data, backed by huge amount of resources.
Your data scale is much less, but your resource provision is also much more limited.
Incremental processing is needed upon the following conditions:
-
High freshness requirement
For most user-facing applications this is needed. e.g. users update their documents,
and it's unexpected if they see stale information in search results.
If the search result is fed into an AI agent, it may mean unexpected response to users (i.e. LLM generate output based on inaccurate information).
It's more dangerous and users may even take the unexpected response without noticing.
-
Transformation cost is significantly higher than retrieval itself
Overall, say T is your most acceptable staleness. If you don't want to recompute the whole thing repeatedly every cycle of T,
you will need incremental processing more or less.
How CocoIndex does incremental processing, by examples​
We could take a look at a few examples to understand what CocoIndex handles behind the scene for incremental processing.
Example 1: Update a document​
Consider this scenario:
- I have a document. Initially, it's split into 5 chunks, resulting in 5 rows with their embeddings in the index.
- After it's updated. 3 of them are exactly the same as previous. Others are changed.
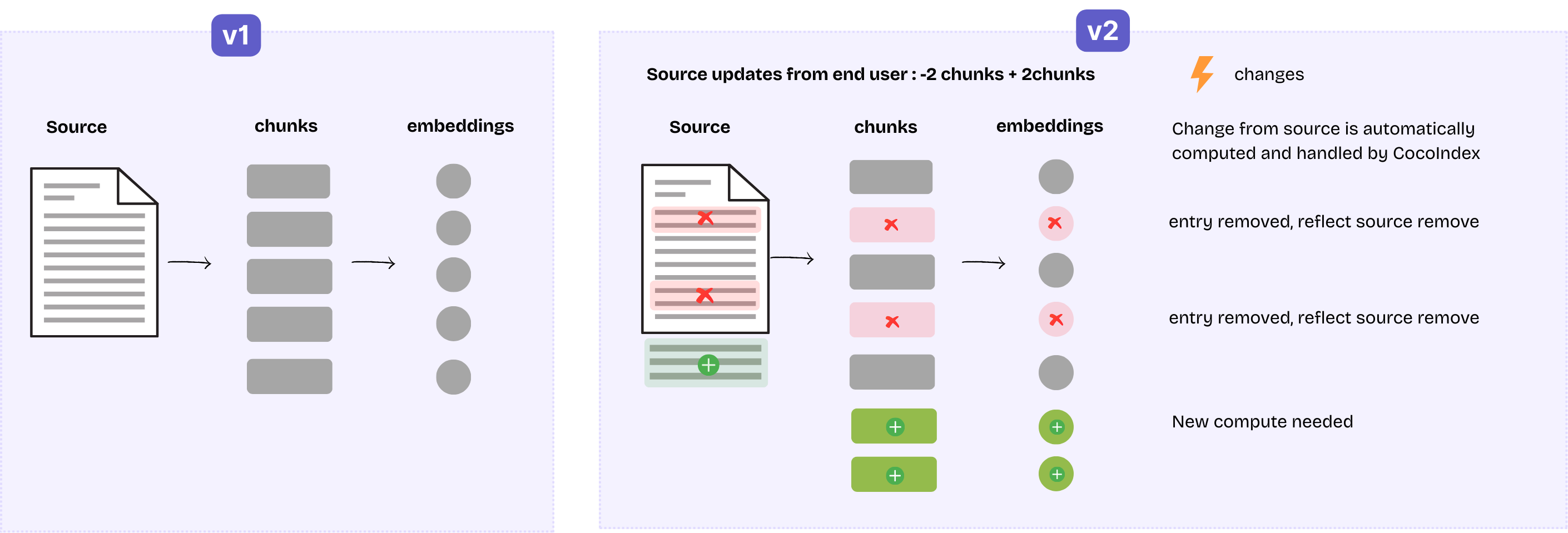
So we need to keep 3 rows, remove 2 previously existing rows, and add 2 new rows. These need to happen behind the scene:
- Ideally, we only recompute embeddings for the 4 new rows, and reuse it for 3 unchanged chunks.
This can save computation cost especially when the embedding API charges by usage.
CocoIndex achieves this by maintaining a cache for heavy steps like embedding, and when the input for a transformation step isn't changed, the output will be reused.
- Besides, we also maintain a lineage tracking in the internal storage.
It keeps track of which rows in the index are derived from the previous version of this document, to make sure stale versions are properly removed.
CocoIndex takes care of this.
Example 2: Delete a document​
Continuing with the same example. If we delete the document later, we need to delete all 7 rows derived from the document.
Again, this needs to be based on the lineage tracking maintained by CocoIndex.
The transformation flow may also be changed, for example, the chunking logic is upgraded, or a parameter passed to the chunker is adjusted.
This may result in the following scenario:
- Before the change, the document is split into 5 chunks, resulting in 5 rows with their embeddings in the index.
- After the change, they become 6 chunks: 4 of previous chunks remain unchanged, the remaining 1 is split into 2 smaller chunks.
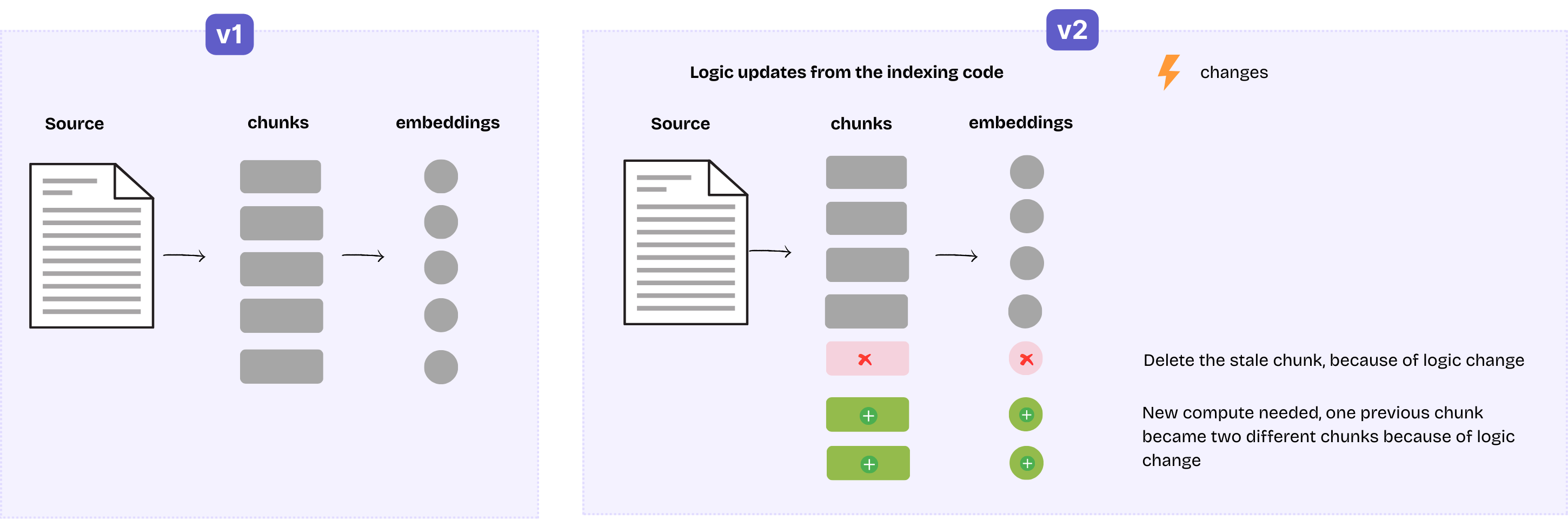
This falls into a similar situation as document update (example 1), and CocoIndex will take care of it. The approach is similar, while this involves some additional considerations:
-
We can still safely reuse embeddings for 4 unchanged chunks by the caching mechanism: this needs a prerequisite that the logic and spec for embedding is unchanged.
If the changed part is the embedding logic or spec, we will recompute the embeddings for everything.
CocoIndex is able to see if the logic or spec for an operation step is changed from the cached version, by putting this additional information in the cache key.
-
To remove stale rows in the target index, the lineage tracking works well again.
Note that some other systems handle stale output deletions on source update/deletion by replaying the transformation logic on the previous version of input:
this only works well when transformation is fully deterministic and never upgraded.
CocoIndex's lineage tracking based approach doesn't have this limitation: it's robust to transformation logic non-determinism and changes.
All examples above are simple cases: each single input row (e.g. a document) is involved independently during each specific transformation.
CocoIndex is a highly customizable framework, not only limited to simple chunking and embedding.
It allows users for more complex advanced transformations, such as:
-
Merge.
For example, you're building an index for "all AI products", and you want to combine information from multiple sources, some product exists in one source and some in multiple.
For each product, you want to combine information from different sources.
-
Lookup.
For example, you also have a data source about company information.
During your transformation for each product, you want to enrich it with information of the company building the product, so a lookup for the company information is needed.
-
Clustering.
For example, you want to cluster different products into scenarios, and create cluster-level summaries based on information of products in the cluster.
The common theme is that during transformation, multiple input rows (coming from single or multiple sources) need to be involved at the same time.
Once a single input row is updated or deleted, CocoIndex will need to fetch other related rows from the same or other sources.
Here which other rows are needed is based on which are involved in the transformations.
CocoIndex keeps track of such relationships, and will fetch related rows and trigger necessary reprocessings incrementally.
Change Data Capture (CDC)​
1. When source supports push change​
Some source connectors support push change.
For example, Google Drive supports drive-level changelog and sends change notifications to your public URL,
which is applicable for team drive and personal drive (only by OAuth, service account not supported).
When a file is created, updated, or deleted, CocoIndex could compute based on the diff.
- Google drive change log is only supported on drive level, not file/folder level.
- With service accounts, you can only enable changelog for team drives, by sharing the team drive with it.
All source connectors in CocoIndex provide a basic list API capability, which enables a generic change detection mechanism applicable to any data source.
For example, with local file systems, we can traverse all directories and subdirectories recursively to get the full list of entries and their metadata (like modification time). By comparing the current state with the previous state, CocoIndex can detect changes even without source-specific change notifications.
This approach works universally across all data sources, though when the number of entries is large, performing a complete traversal can be resource-intensive.
This is a generic mechanism applicable to all data sources, so all data sources can leverage this even if there's no source-specific change capture.
3. Optimizations for specific sources​
When source connector provides advanced features to list entries, for example, list most recently changed entries.
CocoIndex could take advantage of that to provide more efficient change detection.
For example, when changelog is not available for Google Drive, see condition here on when it is not available.
CocoIndex could monitor the change based on last modified vs last poll time, periodic trigger to check modified entries.
However this cannot capture full change, for example when an entry has been deleted.
In CocoIndex, every piece of the lego block in the pipeline can be cached.
Currently, whether or not cache is enabled is decided by the implementation of the function.
For builtin functions, if it performs heavy transformation, cache is enabled.
Custom functions can take a parameter cache.
When True
, the executor will cache the result of the function for reuse during reprocessing.
We recommend to set this to True
for any function that is computationally intensive.
Output will be reused if all these are unchanged: spec (if exists), input data, behavior of the function.
For this purpose, a behavior_version
needs to be provided, and should increase on behavior changes.
For example, this enables cache for a standalone function:
@cocoindex.op.function(cache=True, behavior_version=1)
def compute_something(arg1: str, arg2: int | None = None) -> str:
...
This enables cache for a function defined by a spec and an executor:
class ComputeSomething(cocoindex.op.FunctionSpec):
...
@cocoindex.op.executor_class(cache=True, behavior_version=1)
class ComputeSomethingExecutor:
spec: ComputeSomething
...
Thanks for reading and we love to hear from you!
If you like our work, it would mean a lot to us if you could support Cocoindex on Github with a star.